The more I explore Google Cloud's endless catalog of cloud services, the more I like Google Cloud. This is why before moving forward, I'd like to be transparent that this blog has become little more than thinly veiled Google propaganda, where I will henceforth bombard you with persuasive and subtle messaging to sell your soul to Google. Let's be honest; they've probably simulated it anyway.
It should be safe to assume that you're familiar with AWS Lambda Functions by now, which have served as the backbone of what we refer to as "serverless." These cloud code snippets have restructured entire technology departments, and are partially to blame for why almost nobody knows enough basic Linux to configure a web server or build anything without a vendor. Google Cloud Functions don't yet serve all the use cases that Lambda functions cover, but for the cases they do cover, they seem to be taking the lead.
Lambdas vs Cloud Functions
First off, let's talk about a big one: price. AWS charges based on Lambda usage, whereas Google Cloud Functions are free. The only exception to this is when you break 2 million invocations/month, at which point you'll be hemorrhaging as ghastly 40 cents per additional million. That's ridiculous. I think we've just discovered Google Cloud's lead generation strategy.
What about in terms of workflow? AWS holds an architecture philosophy of chaining services together, into what inevitably becomes a web of self-contained billable items on your invoice. An excellent illustration of this is a post on common AWS patterns which provides a decent visual of this complexity, while also revealing how much people love this kind of stuff, as though SaaS is the new Legos. To interact with a Lambda function in AWS via HTTP requests, you need to set up an API Gateway in front, which is arguably a feat more convoluted and complicated than coding. Pair this with an inevitable user permission struggle to get the right Lambda roles set up, and you quickly have yourself a nightmare- especially if you're trying to get a single function live. Eventually, you’ll get to write some code or upload a horrendous zip file like some neanderthal (friendly reminder: I am entirely biased).
Cloud Functions do have their drawbacks in this comparison. Firstly, we cannot build APIs with Cloud Functions- in fact, without Firebase, we can't even use vanity URLs.
Another huge drawback is Cloud Functions cannot communicate with Google's relational database offering, Cloud SQL. This is big, and what's worse, it feels like an oversight. There are no technical constraints behind this, other than Google hasn't created an interface to whitelist anything other than IP addresses for Cloud SQL instances.
Lastly, we cannot create a fully-fledged API available to be sold or distributed. The is currently no Google Cloud API Gateway equivalent.
Deploying a Cloud Function
By the end of this tutorial we'll have utilized the following Google Cloud services/tools:
- A new Google Cloud Function
- A Google Source Repository to sync to our Github repo and auto-deploy changes.
- A CRON job to run our function on a schedule, via Google Cloud Scheduler.
- The gcloud CLI to enable us to work locally.
You'll notice we lack any mentions of API endpoints, methods, stages, or anything related to handling web requests. It should not be understated that Cloud Functions are preconfigured with an endpoint, and all nonsense regarding whether endpoints accept GET or POST or AUTH or OPTIONs is missing entirely. These things are instead handled in the logic of the function itself, and because Google Cloud functions running Python are preconfigured with Flask, all of that stuff is really trivially easy. That's right, we've got Flask, Python, and GCP all in a single post. Typing these words feels like eating cake while Dwyane The Rock Johnson reads me bedtime stories and caresses me as I fall asleep. It's great.
In the Cloud console, go ahead and create a new function. Our function will take the form of an HTTP endpoint:
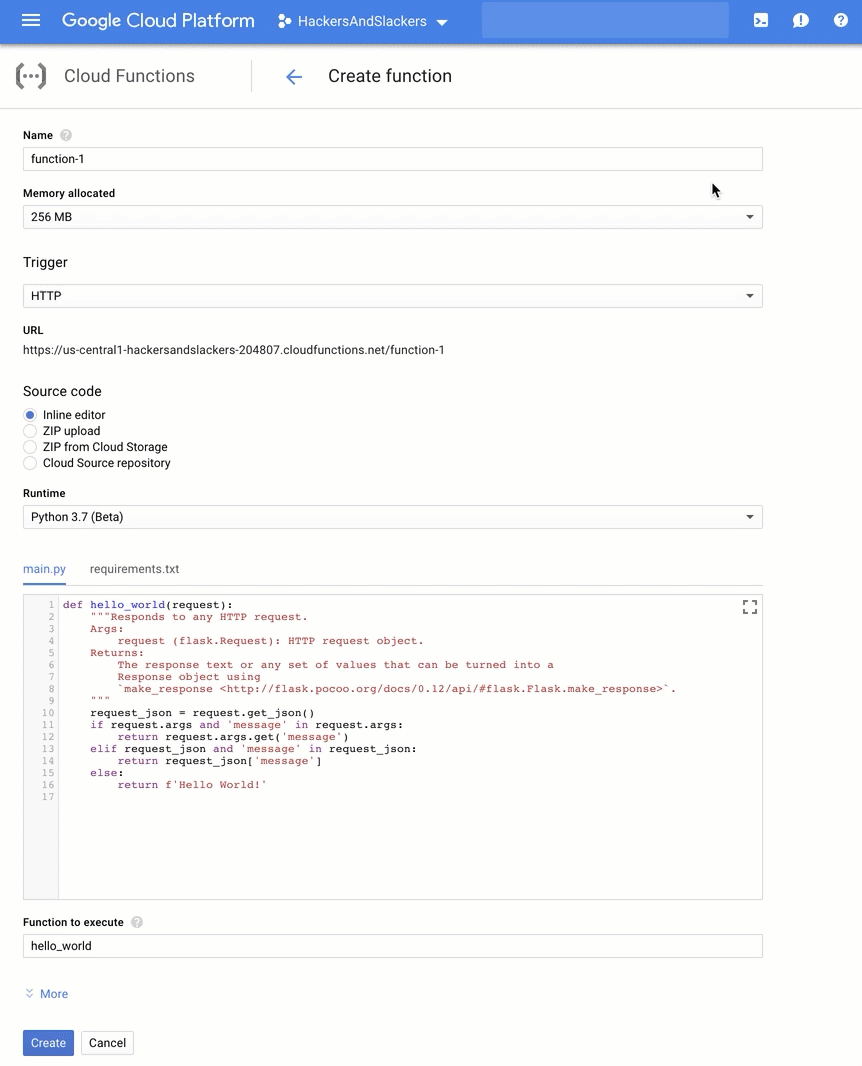
- Memory Allocated lets us allocate more than the default 256MB to our function. Remember that Cloud functions are free: choose accordingly.
- Trigger specifies what will have access to this function. By selecting HTTP, we will immediately receive a URL.
- Source code gives us a few options to deploy our code, with cloud source repository being by far the easiest solution (more on that in a bit).
- Runtime allows you to select NodeJS by accident.
- Function to Execute needs the name of our entry point function, which is to be found in
main.py
ormain.js
depending on which language you’ve selected.
If you're familiar with Lambda functions, the choices of an inline code editor or a zip file upload should come as no surprise. Since you're already familiar, I don't need to tell you why these methods suck for any sane workflow. Luckily, we have a better option: syncing our Github repo to a Google Source Repository.
Google Source Repositories
Google Source Repositories are repos that function just like Github or Bitbucket repos. They're especially useful for syncing code changes from a Github repo, and setting up automatic deployments on commit.
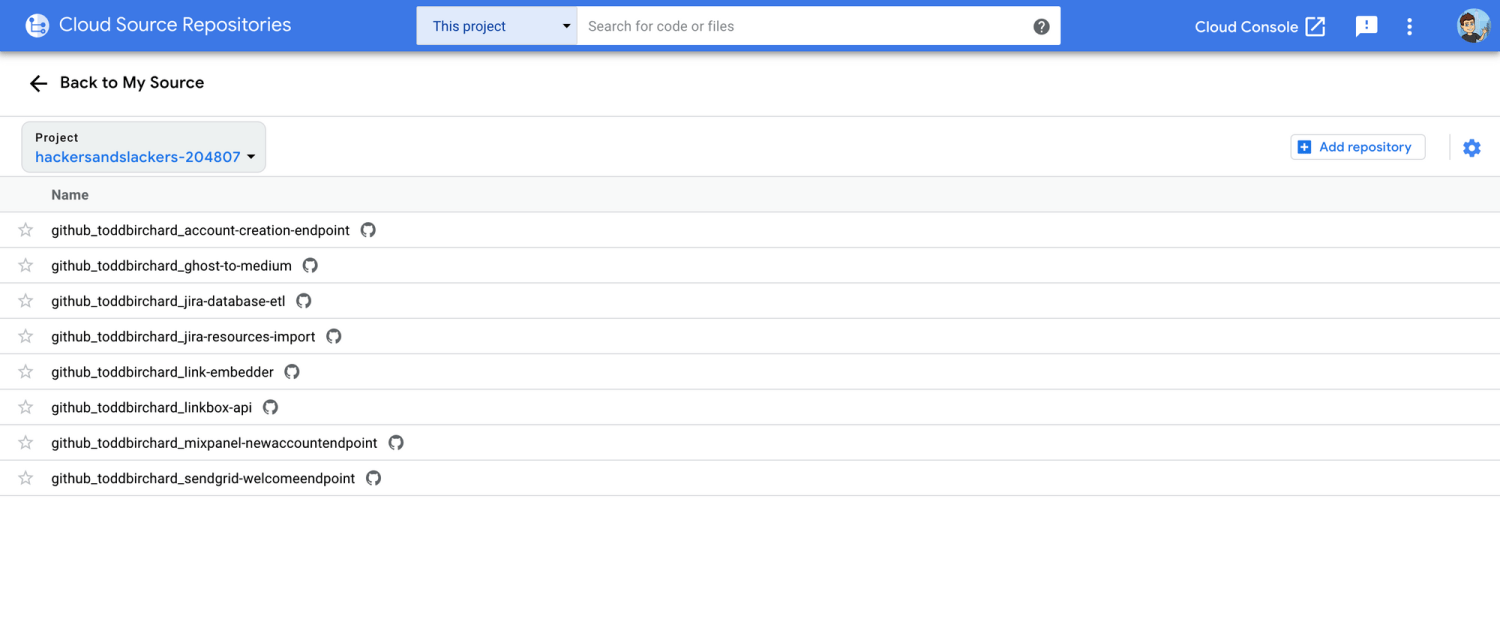
Setting up this sync is super easy. Create a new repository, specify that we're connecting an external Github repo, and we'll be able to select any repo in our account via the GUI:
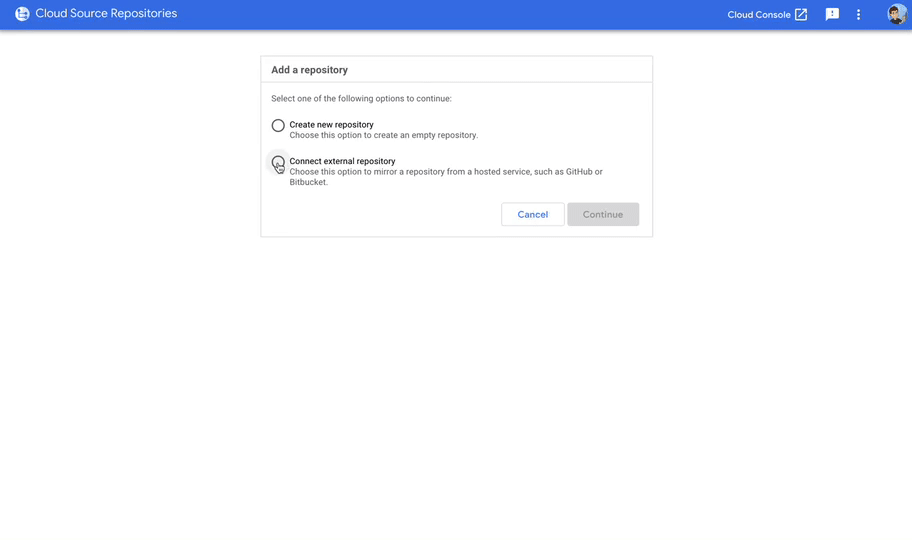
Now when we go back to our editing our function, setting the Source code field to the name of this new repository will automatically deploy the function whenever a change is committed. With this method, we have effectively zero changes to our normal workflow.
Commit to Google Source Repositories Directly
If you don't want to sync a Github repo, no problem. We can create a repo locally using the gcloud CLI:
$ gcloud source repos create real-repo
$ cd myproject/
$ git init
--------------------------------------------------------
(take a moment to write or save some actual code here)
--------------------------------------------------------
$ git add --all
$ git remote add google https://source.developers.google.com/p/hackers/r/real-repo
$ git commit -m 'cheesey init message'
$ git push --all google
Now make that puppy go live with gcloud functions deploy totally-dope-function
, where totally-dope-function is the name of your function, as it should be.
With our function set up and method in place for deploying code, we can now see how our Cloud Function is doing.
Viewing Error Logs
Because we have a real endpoint to work with, we don't need to waste any time creating dumb unit tests where we send fake JSON to our function (real talk though, we should always write unit tests).
The Cloud Function error log screen does a decent job of providing us with a GUI to see how our deployed function is running, and where things have gone wrong:
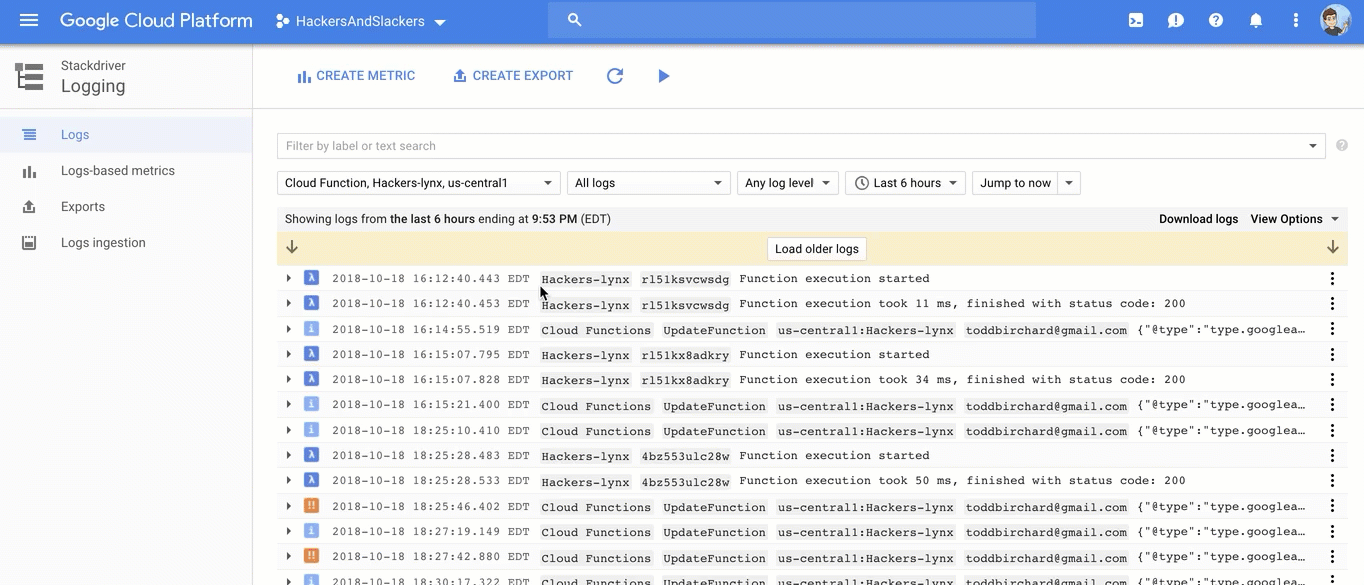
Firing Our Function on a Schedule
Let's say our function is a job we're looking to run daily, or perhaps hourly. Google Cloud Scheduler is a super-easy way to trigger functions via CRON.
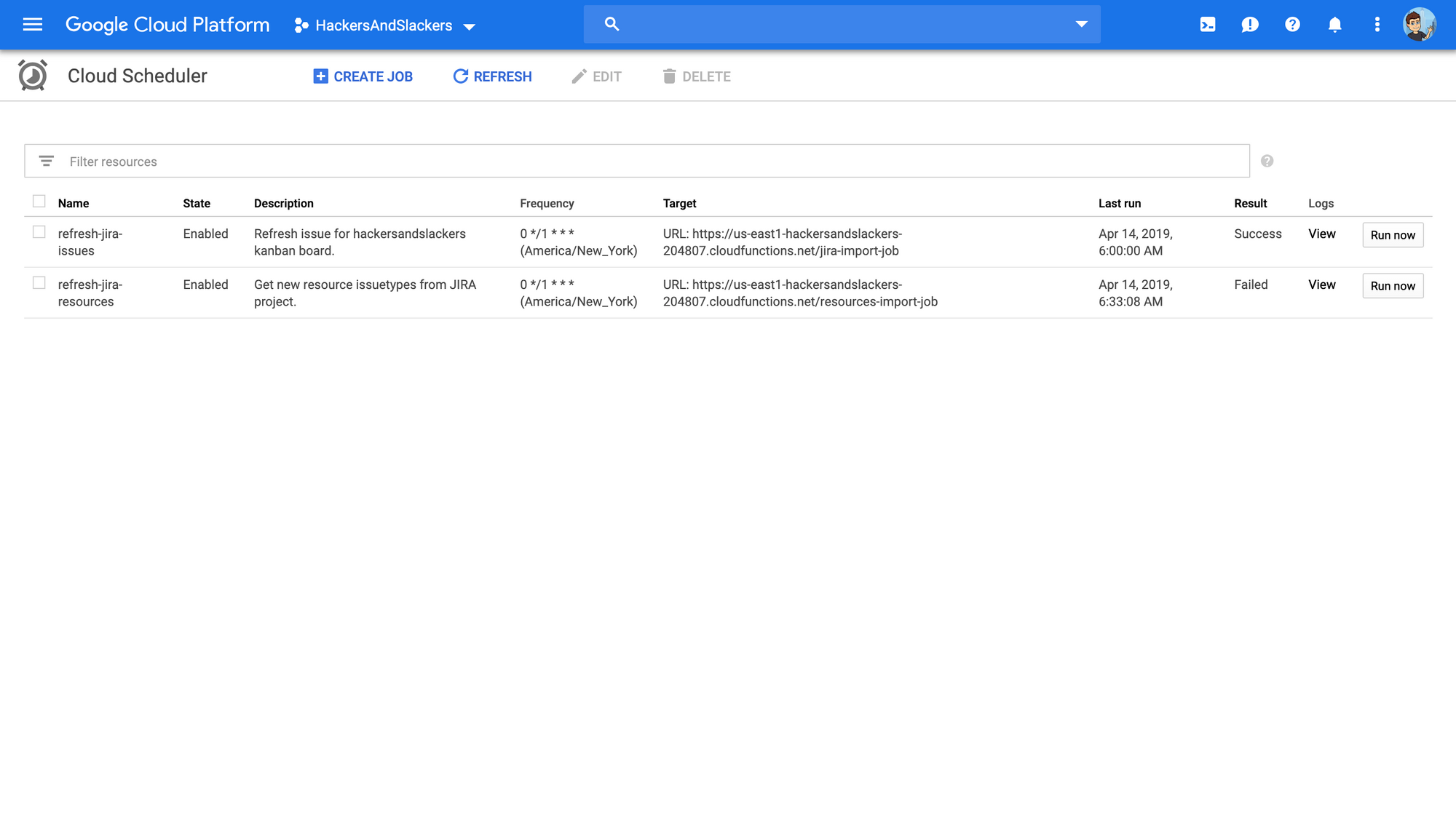
The easiest way to handle this is by creating our function as an HTTP endpoint back when we started. A Cloud Scheduler job can hit this endpoint at any time interval we want - just make sure you wrote your endpoint to handle GET requests.
Cloud Functions in Short
GCP seems to have been taking notes on the sidelines on how to improve this process by removing the red tape around service setup or policy configuration. AWS and GCP are tackling opposites approaches; AWS allows you to build a Robust API complete with staging and testing with the intent that some of these APIs can even be sold as standalone products to consumers. GCP takes the opposite approach: cloud functions are development services for developers. That should probably cover the vast majority of use cases anyway.