In terms of technical ingenuity, Google BigQuery is probably the most impressive data warehouse on the market. BigQuery differs from other data warehouses in that the underlying architecture is shared by everybody on Google Cloud, meaning you don't need to pay for a dedicated cluster of expensive servers to occasionally run queries for large-scale data analysis.
Moving data into data warehouses traditionally involves dumping a shit ton of unstructured or semi-structured files into storage such as S3, Google Storage, or data lakes before loading them to their destination. It's no surprise that generations inspired by MapReduce perpetuate outdated processes in technology, as fans of Java typically do (don't @ me). Google upheld this status quo with BigQuery, which we painfully worked through in a previous tutorial. Luckily there are mysterious heroes among us, known only as "third-party developers."
PyBigQuery is a connector for SQLAlchemy, which allows us to connect to and query a BigQuery table as though it were a relational database, as you've certainly done with either PyMySQL or Psycopg2. It really is as easy as it sounds. To make things interesting, we’re going to build a small script to pipe data back and forth from BigQuery to MySQL, and we’ll do it by creating instances of a database-flavor-agnostic class for both sides. Now we’re getting saucy.
DISCLAIMER: Just because we can interact with BigQuery as easily as an ACID-compliant relational database doesn't mean we always should. It's your responsibility to understand the pros and cons of data warehouses and recognize when to use them.
Making our First Big Query
Let's get you set up to do the bare minimum: pulling rows from BigQuery with SQLAlchemy. I’ll save us the agony of explaining what a gcloud credentials JSON file is and assume you already have one for your project.
The obvious Python packages we should install are PyBigQuery and SQLAlchemy. Remember: we're not only going to easily connect to BigQuery, but also demonstrate how easy it is to ship information back and forth between this modern data warehouse and traditional relational databases. In my case, I'm stuck using MySQL:
$ pip3 install pybigquery pymysql sqlalchemy loguru
Just like normal SQLAlchmey connections, we need to create a connection string URI for connecting to BigQuery. You'll notice some new terminology below:
bigquery://[GOOGLE_CLOUD_PROJECT_NAME]/[BIGQUERY_DATASET_NAME]
Never fear- you can easily find your GCP project name, BigQuery Dataset Name, and BigQuery Table Name via the Bigquery console:
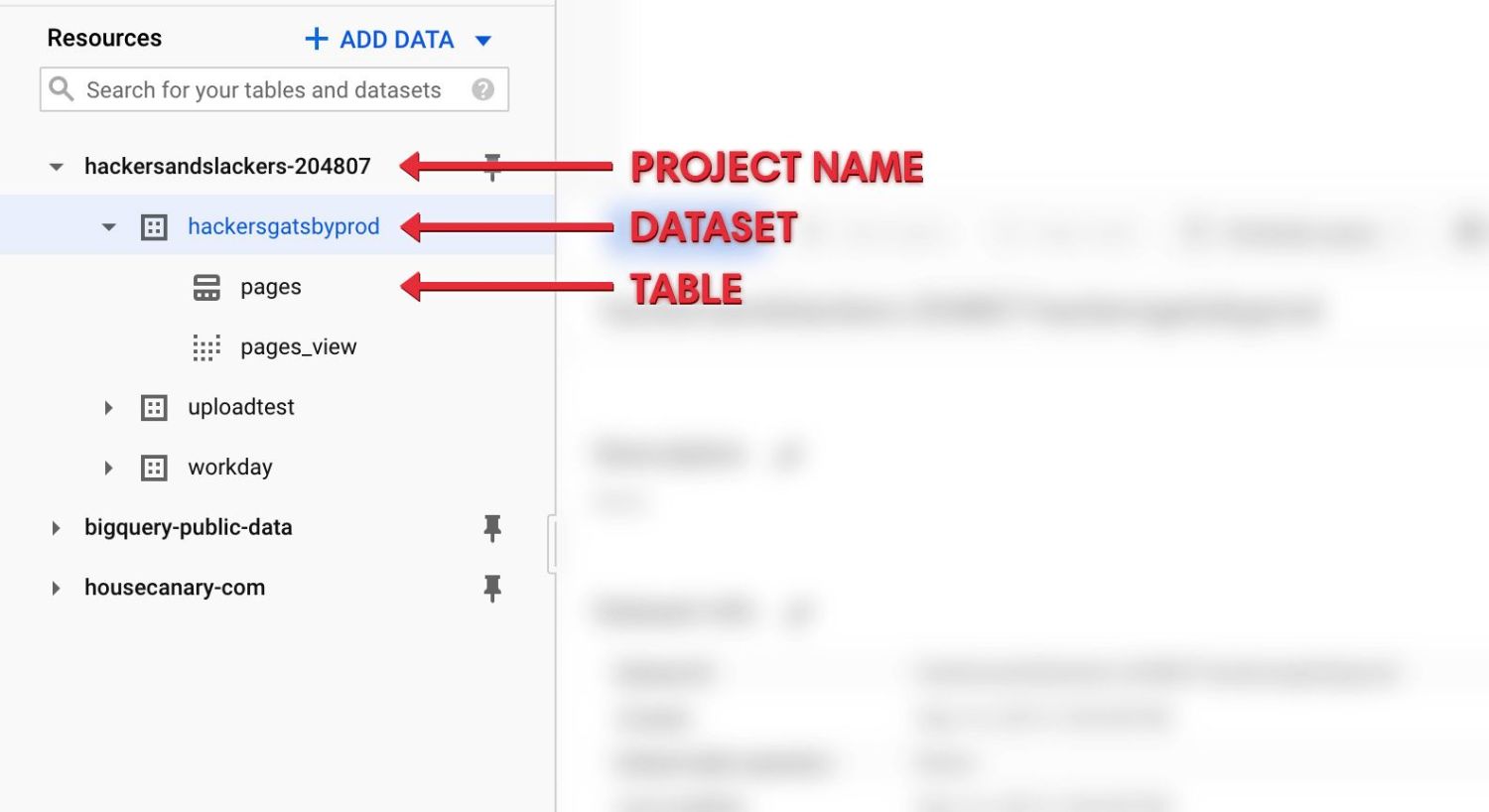
Toss all of this goodness into a config file like the one I have below. As an added bonus, construct your bigquery_uri
variable here to avoiding passing tons of config values later:
"""BigQuery Configuration."""
from os import environ
# Google BigQuery config
gcp_credentials = environ.get('GCP_CREDENTIALS')
gcp_project = environ.get('GCP_PROJECT')
bigquery_dataset = environ.get('GCP_BIGQUERY_DATASET')
bigquery_table = environ.get('GCP_BIGQUERY_TABLE')
bigquery_uri = f'bigquery://{gcp_project}/{bigquery_dataset}'
Connecting to BigQuery
Creating an SQLAlchemy engine for BigQuery should look the same as always, with the only notable difference being the credentials_path parameter now points to our gcloud credentials JSON file:
from sqlalchemy.engine import create_engine
from config import bigquery_uri
engine = create_engine(
bigquery_uri,
credentials_path='/path/to/credentials.json'
)
We can now hit BigQuery as we would with any SQLAlchemy engine:
...
query = f'SELECT title, url, referrer FROM {bigquery_dataset}.{bigquery_table} \
WHERE referrer IS NOT NULL \
AND title IS NOT NULL \
ORDER BY RAND () LIMIT 20;'
rows = engine.execute(query).fetchall()
The rows variable returned by our query is a typical SQLAlchemy ResultProxy, which makes it easy to pass this data to other destinations. We can preview the data we received just for fun:
...
rows = [dict(row) for row in rows]
pp = pprint.PrettyPrinter(indent=2)
pp.pprint(rows)
And here it is:
[
{
"referrer": "https://www.google.com/",
"title": "The Art of Routing in Flask",
"url": "https://hackersandslackers.com/the-art-of-building-flask-routes/"
},
{
"referrer": "https://www.facebook.com/",
"title": "Demystifying Flask\"s Application Factory And the Application Context",
"url": "https://hackersandslackers.com/flask-application-factory/"
},
{
"referrer": "https://www.google.com/",
"title": "Connect Flask to a Database with Flask-SQLAlchemy",
"url": "https://hackersandslackers.com/manage-database-models-with-flask-sqlalchemy/"
},
{
"referrer": "https://www.google.com/",
"title": "Constructing Database Queries with SQLAlchemy",
"url": "https://hackersandslackers.com/constructuing-database-queries-with-the-sqlalchemy-orm/"
},
{
"referrer": "https://www.google.com/",
"title": "Constructing Database Queries with SQLAlchemy",
"url": "https://hackersandslackers.com/constructuing-database-queries-with-the-sqlalchemy-orm/"
}
]
ETL with BigQuery & SQL Databases
If pulling rows from BigQuery was all you hoped to accomplish, feel free to skip away happily. For those ambitious few who remain, I'll let you in on a secret: this is not a 5-liner "trick" tutorial. Oh, no... this tutorial is about building the groundwork for an unstoppable data machine. This is a mission to liberate tabular data to move freely from data warehouse to database, unaware of the borders that once stood between them. We're building the opposite of Brexit.
As the SQLAlchemy community grows, a new landscape begins to unfold where every data source and destination in our stack is compatible with SQLAlchemy. It might not sound astounding, but the idea of data sources sharing a single dialect is somewhat unprecedented. Forget about Spark clusters or messaging queues: we're going to standardize how we work with every data source. We'll do this by abstracting databases via Python classes, as such that fetching rows from MySQL would use the same method as fetching from Postgres or BigQuery without any idiosyncrasies.
Classes are in Session
I'm going to create a single Python class flexible enough to connect to any source. This class will have methods to fetch or insert rows, so we can easily use the same class for each data source, regardless of which is the origin of data versus the recipient. Our class has three notable methods:
fetch_rows()
: Executes a query intended to SELECT rows.insert_rows()
: Accepts a collection of rows to be forced down the throat of a database table. We can chose to replace the existing table if one exists by using the replace keyword arg.construct_response()
Creates a nice human-friendly synopsis of the end result of our data's pilgrimage.
Quite simple stuff:
"""Generic Data Client."""
from sqlalchemy import MetaData, Table
class DataClient:
def __init__(self, engine):
self.engine = engine
self.metadata = MetaData(bind=self.engine)
self.table_name = None
@property
def table(self):
if self.table_name:
return Table(self.table_name, self.metadata, autoload=True)
return None
def fetch_rows(self, query, table=None):
"""Fetch all rows via query."""
rows = self.engine.execute(query).fetchall()
return rows
def insert_rows(self, rows, table=None, replace=None):
"""Insert rows into table."""
if replace:
self.engine.execute(f'TRUNCATE TABLE {table}')
self.table_name = table
self.engine.execute(self.table.insert(), rows)
return self.construct_response(rows, table)
@staticmethod
def construct_response(rows, table):
"""Summarize results of an executed query."""
columns = rows[0].keys()
column_names = ", ".join(columns)
num_rows = len(rows)
return f'Inserted {num_rows} rows into `{table}` with {len(columns)} columns: {column_names}'
Now we leverage the same DataClient class twice: one per data source/destination. If we take a closer look, we can see that insert_rows()
expects an input of rows, which happens to be the same data type that fetch_rows()
outputs. That means we could "fetch" rows from source A and "insert" those rows into destination B in only two lines of code. Moving data in the opposite direction is just as easy. Isn't it great when we all agree on standard libraries?
We still need to get set up to create a client for our MySQL database, which is no sweat. Let's knock that out, similar to what we just did.
SQL Database Client
Going back to our config.py file, let's add variables for connecting to MySQL:
from os import environ
# Google BigQuery config
gcp_credentials = environ.get('GCP_CREDENTIALS')
gcp_project = environ.get('GCP_PROJECT')
bigquery_dataset = environ.get('GCP_BIGQUERY_DATASET')
bigquery_table = environ.get('GCP_BIGQUERY_TABLE')
bigquery_uri = f'bigquery://{gcp_project}/{bigquery_dataset}'
# SQL database config
rdbms_user = environ.get('DATABASE_USERNAME')
rdbms_pass = environ.get('DATABASE_PASSWORD')
rdbms_host = environ.get('DATABASE_HOST')
rdbms_port = environ.get('DATABASE_PORT')
rdbms_name = environ.get('DATABASE_NAME')
rdbms_uri = f'mysql+pymysql://{rdbms_user}:{rdbms_pass}@{rdbms_host}:{rdbms_port}/{rdbms_name}'
# Locally stored queries
local_sql_folder = 'sql'
We've just expanded on what we had to accommodate for a second data source (we may refer to our SQL database as rdbms every now and then, in case anybody is confused. Now let's create our second engine:
"""SQL Database Engine."""
from sqlalchemy.engine import create_engine
from config import rdbms_uri
rdbms_engine = create_engine(rdbms_uri)
Time to tie it all together.
It Takes Two
Let's tango. First, we create our two data sources:
from biquery_sql_etl.engines import bigquery_engine, rdbms_engine
from biquery_sql_etl.client import DataClient
def init_pipeline():
"""Move data between Bigquery and MySQL."""
bqc = DataClient(bigquery_engine)
dbc = DataClient(rdbms_engine)
Nothing crazy here! bqc is our BigQuery client, and dbc is our relational database client.
Now what sort of data shall we move between the two? I've gone ahead and worried about that on our behalf by importing a bunch of SQL queries I've stored in a dictionary named sql_queries
! If you're interested in why I've stored these as a dictionary, check out the source code at the end.
Here's how it's gonna go: each query is going to select a bunch of rows from BigQuery, and dump those rows into MySQL. At the end, we'll log how many rows were migrated:
...
from loguru import logger
from biquery_sql_etl.queries import sql_queries
logger.add('logs/queries.log', format="{time} {message}", level="INFO")
def init_pipeline():
"""Move data between Bigquery and MySQL."""
num_rows = 0
bqc = DataClient(bigquery_engine)
dbc = DataClient(rdbms_engine)
for table_name, query in sql_queries.items():
rows = bqc.fetch_rows(query)
insert = dbc.insert_rows(rows, table_name, replace=True)
logger.info(insert)
num_rows += len(rows)
logger.info(
f"Completed migration of {num_rows} rows from BigQuery to MySQL."
)
Aaaand the output:
2020-02-11 07:29:26.499 - Inserted 100 rows into `weekly_stats` with 4 columns: title, url, slug, views
2020-02-11 07:29:28.552 - Inserted 500 rows into `monthly_stats` with 4 columns: title, url, slug, views
2020-02-11 07:29:28.552 - Completed migration of 600 rows from BigQuery to MySQL.
What Can We Take From This?
Capitalism tends to consolidate things over time (businesses, products, choices), yet somehow it seems we have more database & data warehouse options than ever. The last decade has brought us Redshift, BigQuery, CockroachDB, Cassandra, Snowflake... the list goes on. The only trend more ridiculous than this abundance of choice is a trend within enterprises to incorporate all of them. I'm willing to bet your mid-sized company has a data stack which includes:
- Multiple types of RDBs
- A data warehouse
- An inexplicably misused DynamoDB instance
- A forgotten MongoDB database that some frontend guy set up years ago and is actively getting hacked into as we speak.
Making decisions is how non-contributors force themselves into office space relevance. More options mean more opportunities for individuals to make self-serving choices. In software teams, this usually translates into nonsensical architecture where each service, database, or data warehouse is a relic of an unseen political play. I don't see this changing anytime soon, so maybe we are on to something after all.
Source code for this tutorial found here: