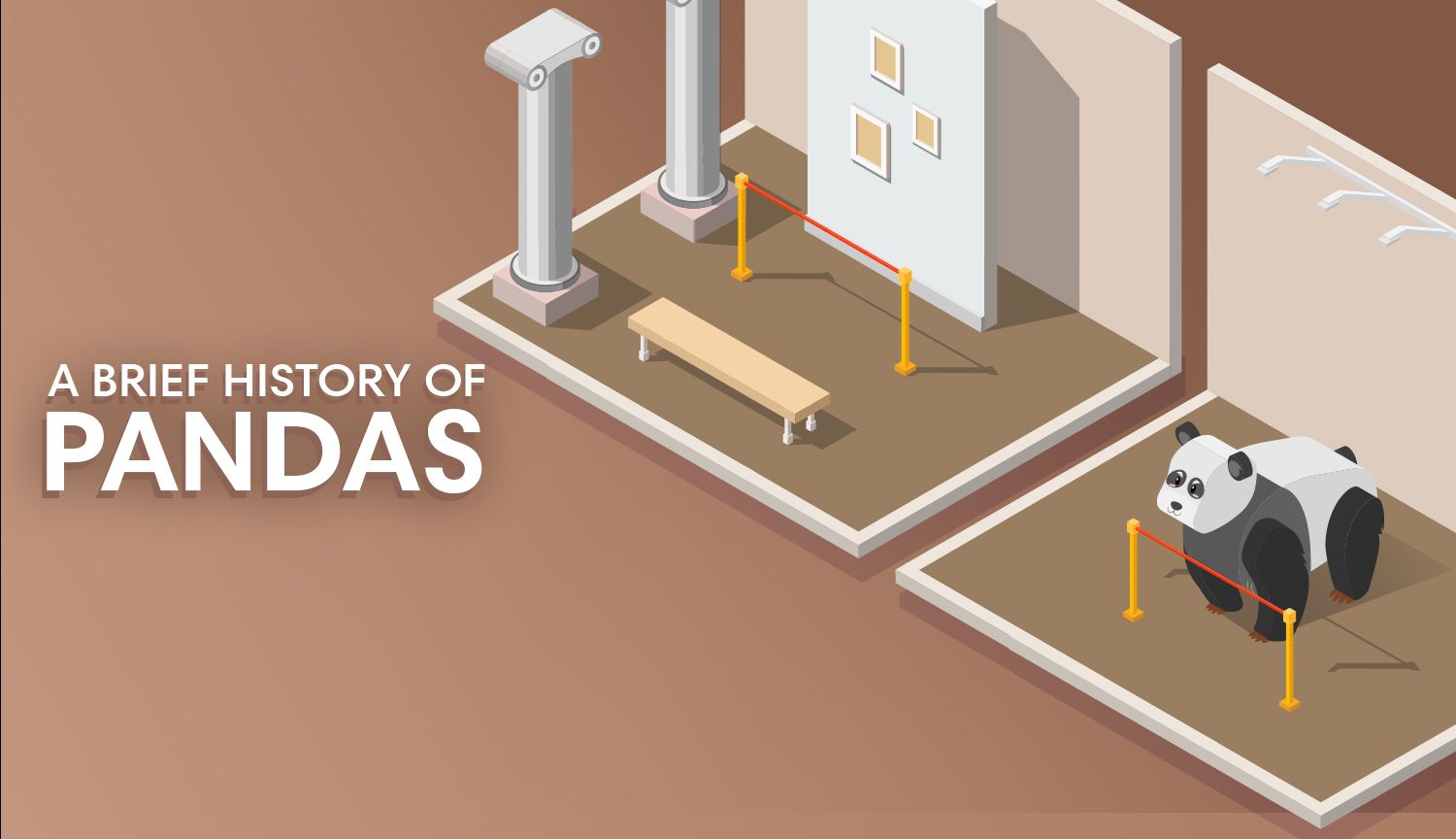
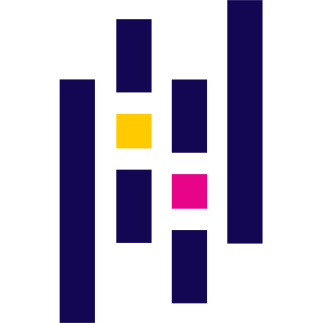
Pandas
Analyze data with the Pandas data analysis library for Python. Start from the basics or see real-life examples of pros using Pandas to solve problems.
Tags
Python
Software
DevOps
Architecture
Data Engineering
Pandas
Excel
Data Analysis
REST APIs
Data Science
SQL
Flask
Code Snippet Corner
JavaScript
AWS
NodeJS
Google Cloud
Frontend
MySQL
Apache
Data Vis
BI
NoSQL
Spark
GraphQL
ExpressJS
PostgreSQL
ETL Pipelines
Tableau
Machine Learning
Big Data
Powerpivot
PowerBI
Atlassian
GatsbyJS
Automation
SQLAlchemy
Data Warehouses
Mapbox
Plotly
Golang
JAMStack
Scraping
Django
Concurrency
ReactJS
SaaS Products
Hashicorp
Docker
Terraform
Frameworks
FastAPI
Java
Microsoft
Newsletter
Create an account to receive occasional updates and interact with the community.